Day one focused mainly around successfully implementing AI. Responsible AI (Trustworthy & Ethical) were topics mentioned during the presentations and discussions. Read a summary of the highlights here: https://www.responsibleai.news/post/data-ai-expo-highlights-of-day-one
Day two focused on Safe, Secure and Responsible AI. Here are some highlights and learnings from four key sessions:
Keynote: Ensuring your AI is responsible and ethical
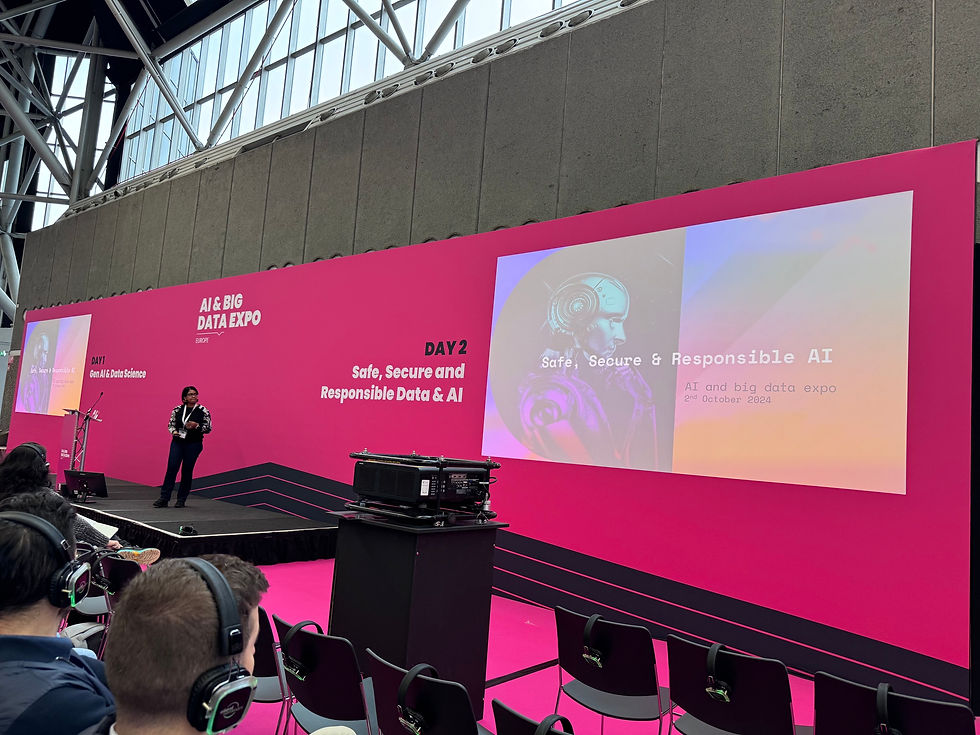
Different interpretations of the EU Act will cause organizations to be unclear what intelligent systems exactly should be regulated
Responsible AI requires detailed understanding of the data
Data should be representative, consistent and accurate
Algorithms should be transparent and explainable
Facial recognition in public
Under EU Act this is not allowed without juridical approval
For example currently done in UK without approval
How can be build ethical responsible systems
What are my beliefs and principles
How do I implement ethical ai
Why important?
Impacts everyone daily, especially minorities
What can you do?
Be conscious about your choice when developing AI
Check data bias, model bias, operational bias
EU AI Act
High risk AI requires risk & quality management system, data governance
Generative AI is not classified as high risk, possibly stronger regulation in the future
High risk AI governance in place by August 2026
Interpretation, implementation and enforcement for AI developers is still unclear
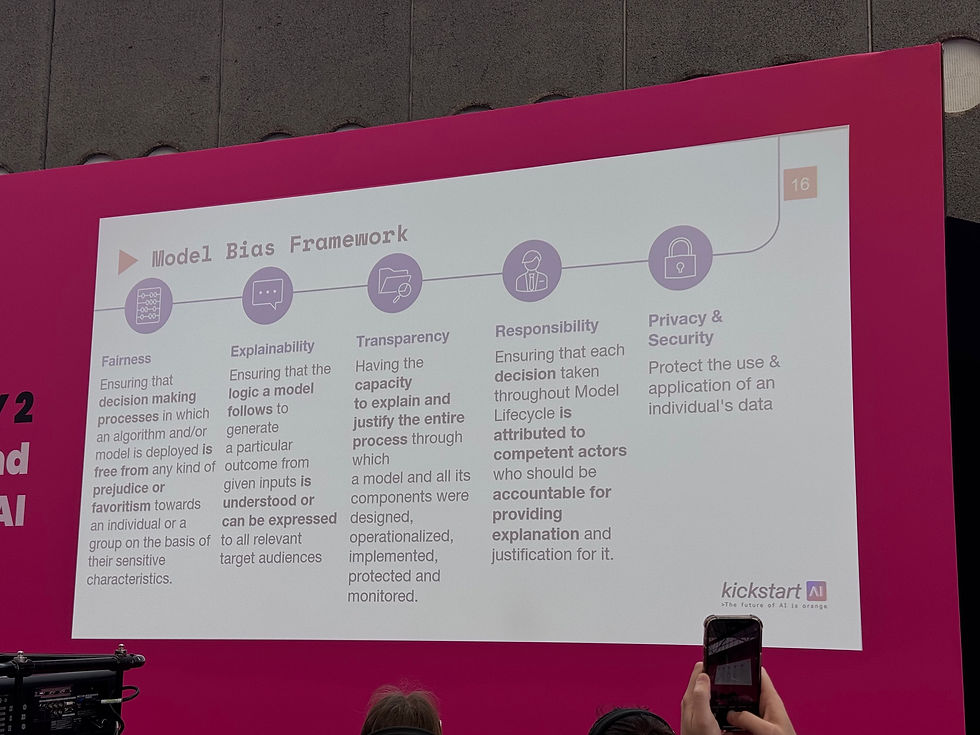
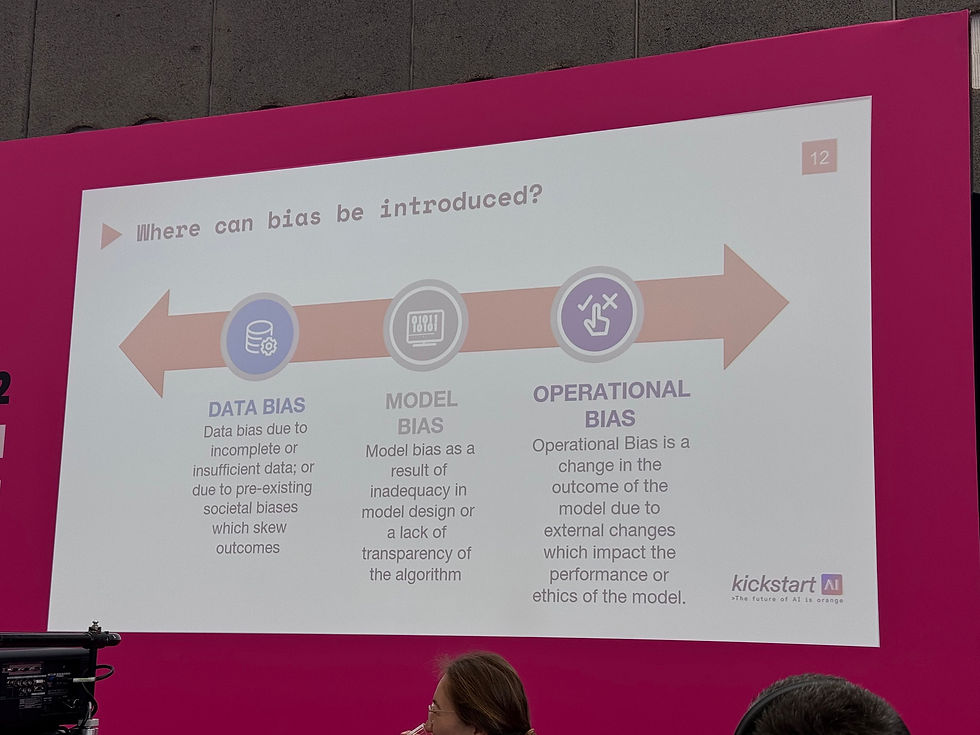
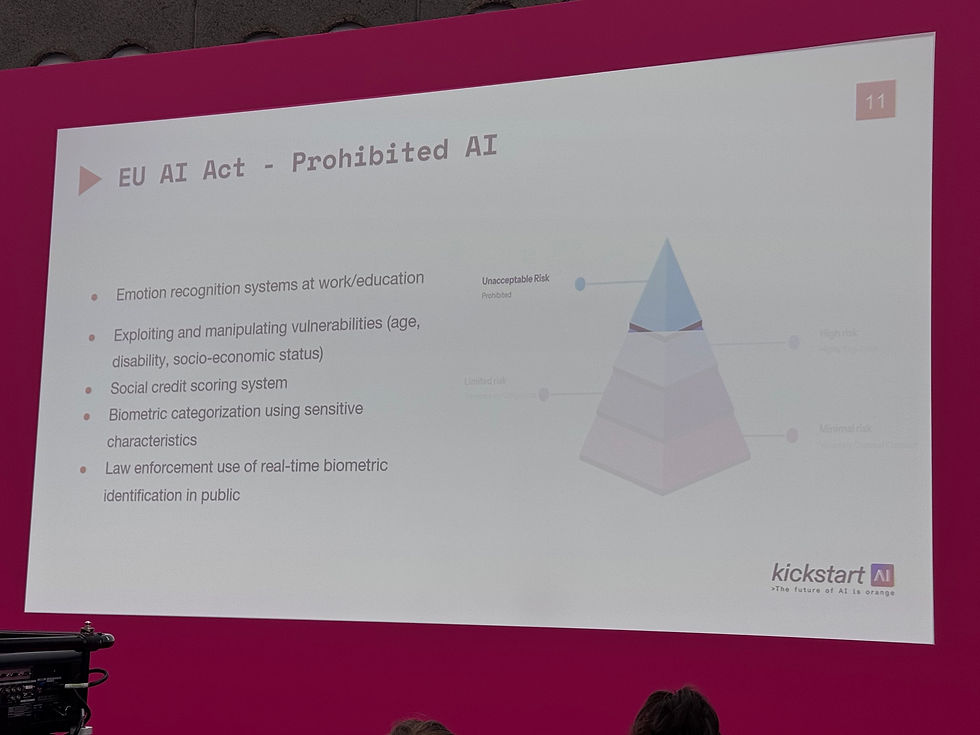
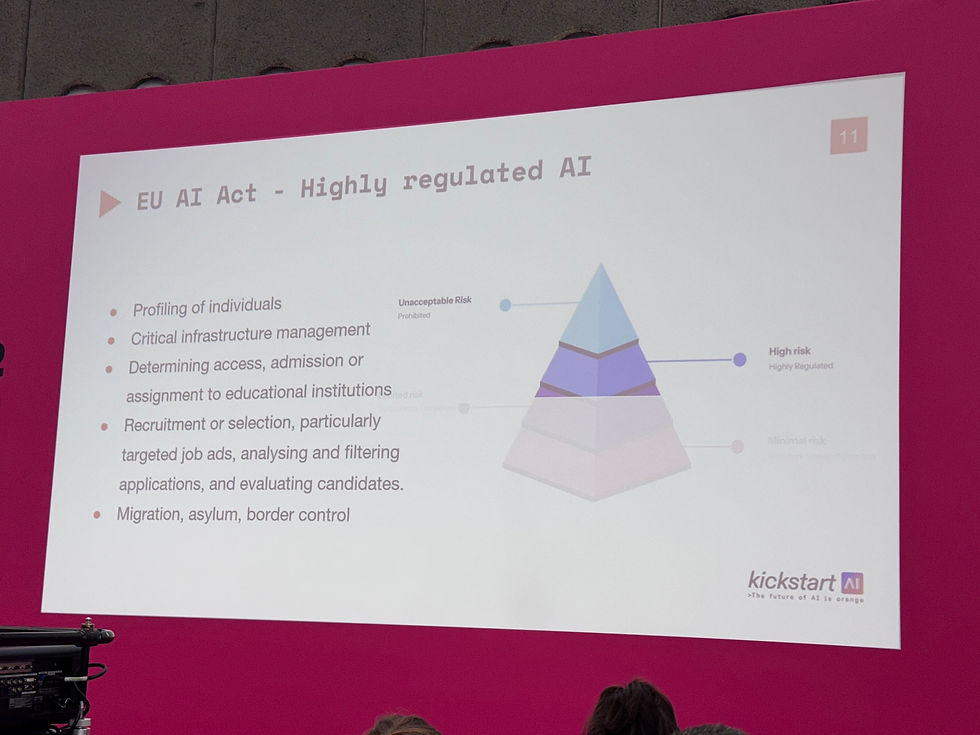
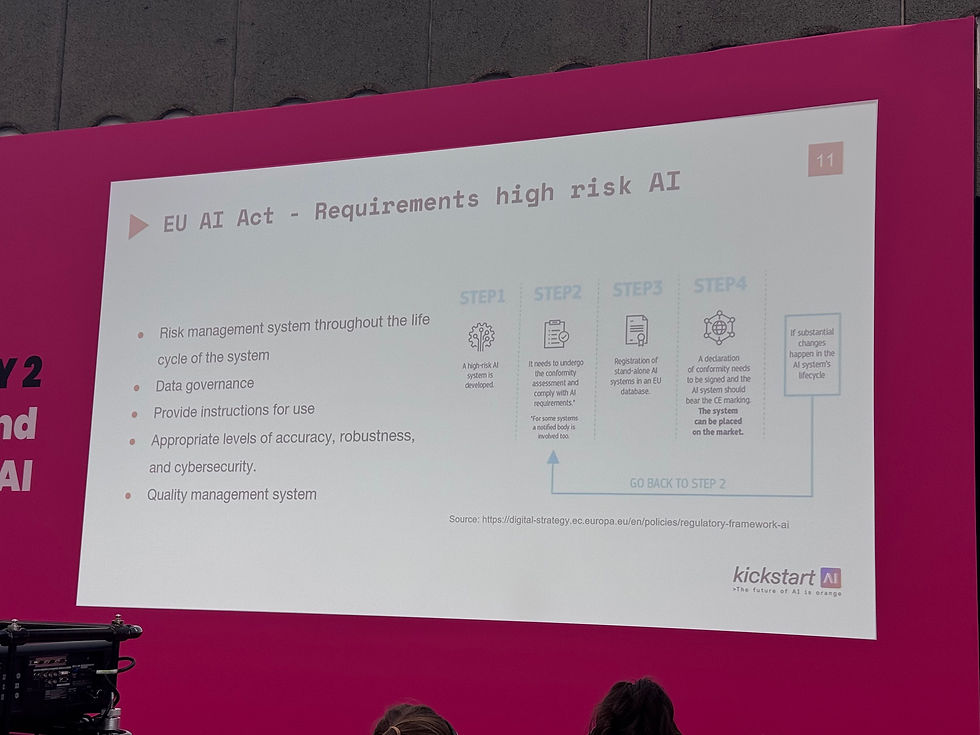
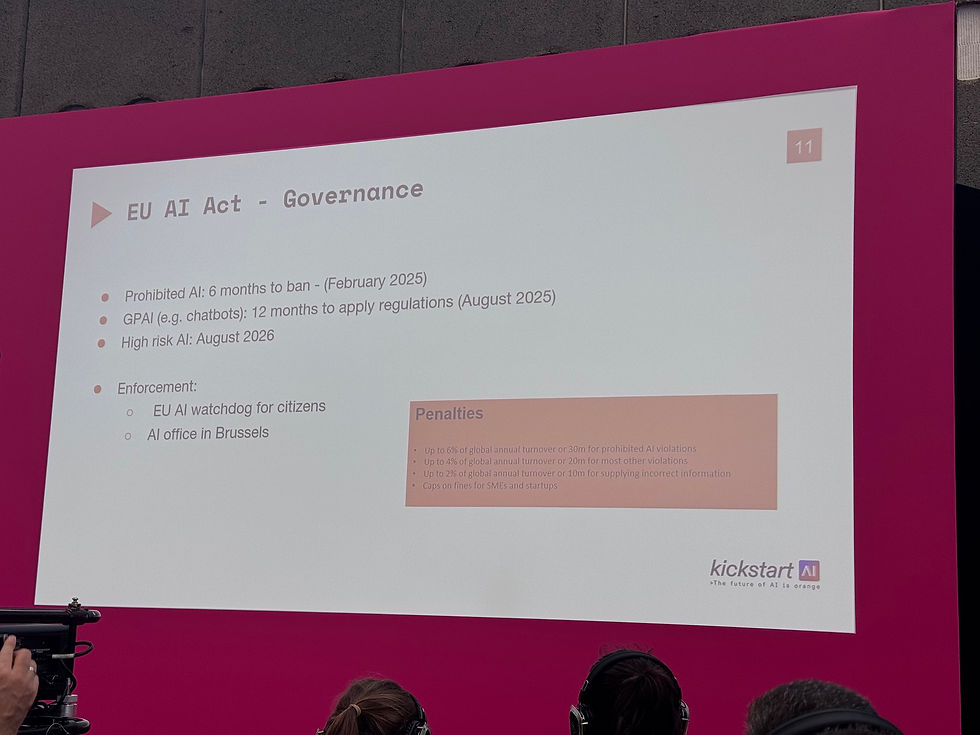
Panel Discussion: Ethical Considerations in Gen AI and Data Science - Navigating Complex Terrain
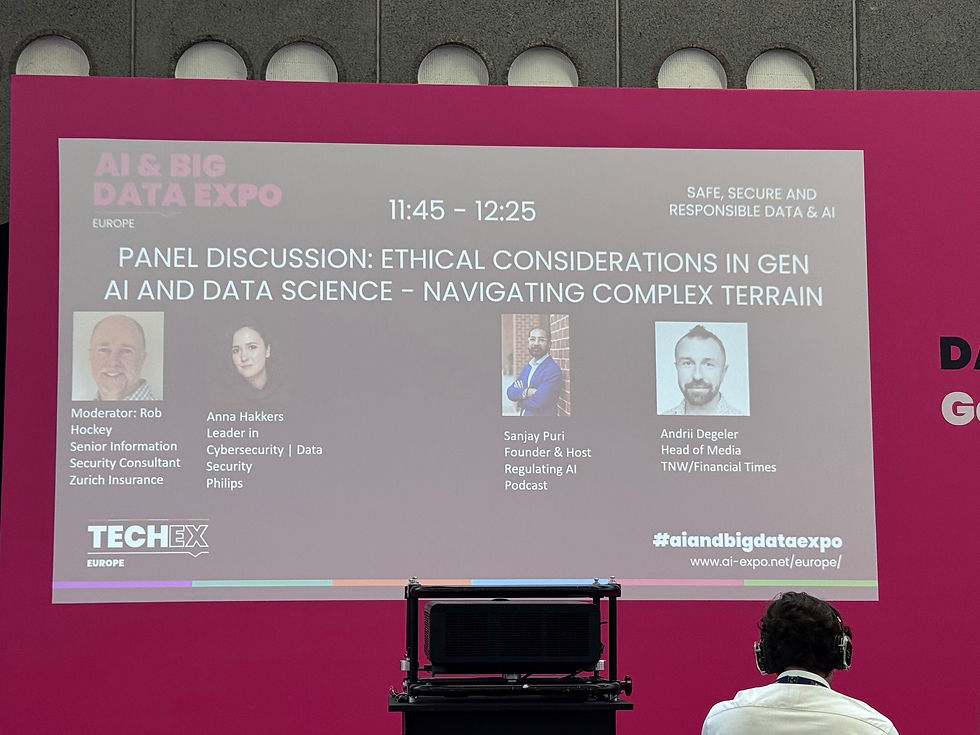
Aspects of EU AI act making biggest impact are
Application and software stack (AI is not just another software) -> security (=data) vulnerability
Transparency and explainability requirements will ensure that users understand how their data is used
Aspects of AI having biggest positive impact
Healthcare (faster detection and more efficient treatment) and pharmaceuticals (faster medicine development)
Impact of AI to Privacy
Depends on the application and personal data usage
Sophisticated algorithms do not need as much personal data as before to make conclusions
Data hungryness of large AI models will impact governance
GenAI impact on disinformation
Scale, speed and accuracy is increasing with AI
When well governed then it could put more power in the hands of the public
Dealing with copyrights
So far not appropriately dealt with, companies avoiding responsibility by using “fair use argument”
Difference in regulation between EU and US (where no comprehensive legislation is planned with some exception for use cases such as deepfakes)
Panel Discussion: Navigating the EU AI Act - Implications and Opportunities for Innovation and Regulation

Between 70-80% of AI project fail (according to Gartner) due to misalignment between people and technology
For organizations to comply to the EU AI Act they need to select the appropriate risk management framework (there are several)
Big challenges expected for small and medium sized companies due to resources and skills needed
Some AI product companies are moving to US due to less regulation (and better investment climate)
Even for lower risk levels e.g. for companies using existing LLMs provided for example by OpenAI or Meta, there are very specific compliance requirements which companies need to become aware of but also become of the cybersecurity risks for example specifically when running an LLM
It is possible to be compliant to EU AI Act but be vulnerable to cyber attacks and loose money (and reputation)
Companies should consider running more specific and local LLMs, train them and run them as a souvereign service
Fastweb in Italy is being trained in Italian language and locally relevant data and provided as a service to Italian companies
Sustainability is also a key topic part of the EU AI Act but currently not getting much attention
Improvement in energy management, hardware (datacenter), networking and model (transporter) innovation is happening
Chicken and Egg Problem: Securing Sensitive Data with and from AI
Sophisticated attacks are not yet happening since they are too costly, simple attacks have better ROI
When start happening they will be very limited / focused
AI security is becoming its own category (are novel) since it has its own specific vulnerabilities
Require novel methods
Cannot be reliably detected and mitigated
Cannot be patched - no do-overs (too costly to re-train)
Challenges today
Use and development of AI is rising
Secure AI is a white spot (can regulations keep up)
Data security
Is different since takes a holistic approach (human, internal, external)
Data breach is very costly (can have big societal impact)
Access to sensitive data and third party software and components
Future
Novel techniques being developed
Standard, guidelines and frameworks
Joint expertise: developers, ai experts, cybersecurity experts
Data security triangle
Visibility: ai inventory, prioritisation
Governance: training, operation, input
Risk analysis: risks, mitigations, gaps
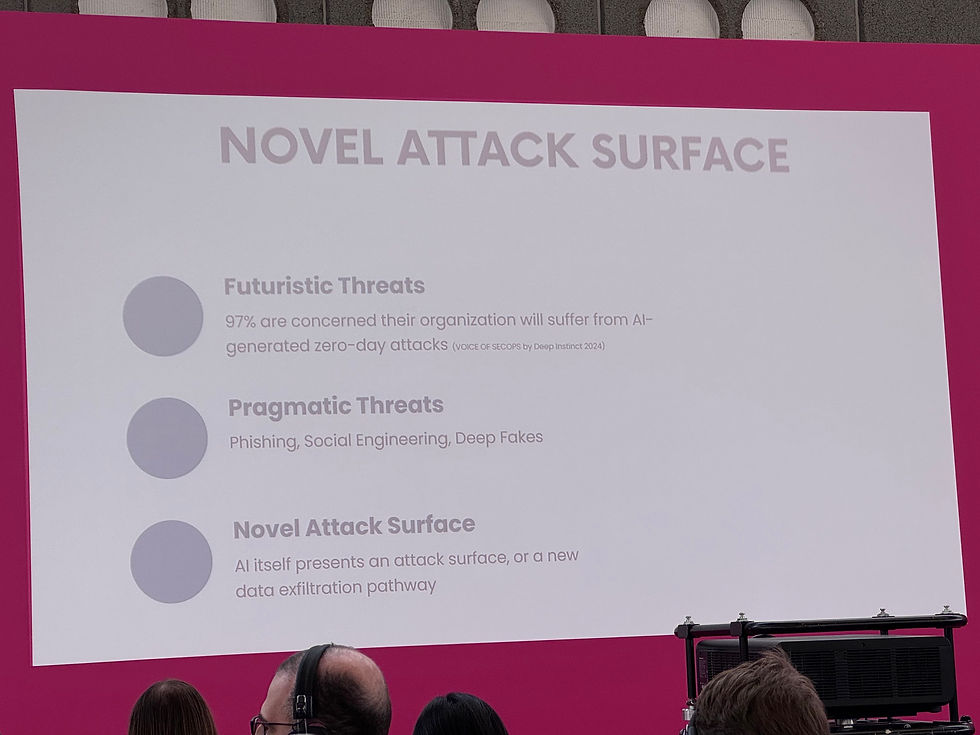
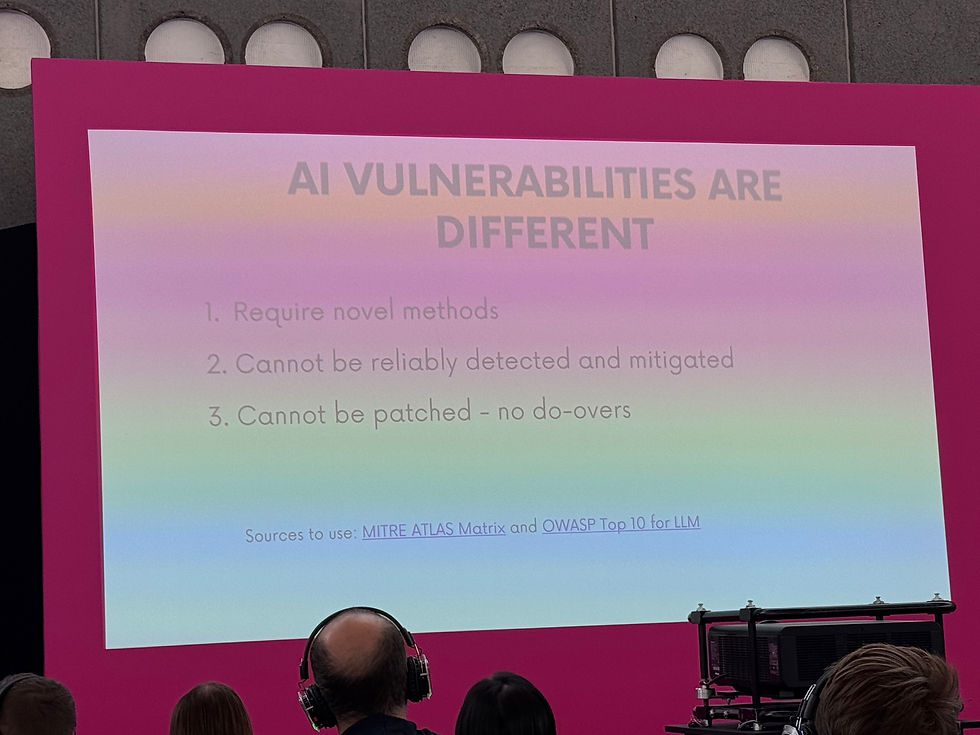
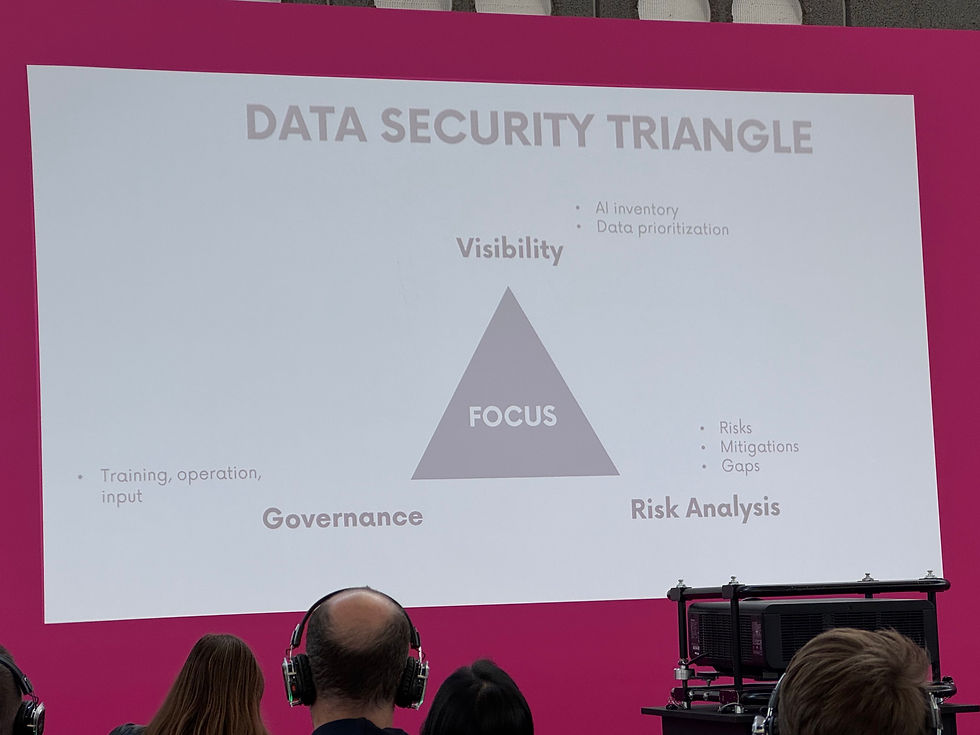
コメント